Document Type
Article
Publication Title
EURASIP Journal on Information Security
Publication Date
2019
Keywords
ARIMA, GARCH, RNN, Hybrid models, LSTM, Deep learning, BRNN-LSTM
Abstract
Like how useful weather forecasting is, the capability of forecasting or predicting cyber threats can never be overestimated. Previous investigations show that cyber attack data exhibits interesting phenomena, such as long-range dependence and high nonlinearity, which impose a particular challenge on modeling and predicting cyber attack rates. Deviating from the statistical approach that is utilized in the literature, in this paper we develop a deep learning framework by utilizing the bi-directional recurrent neural networks with long short-term memory, dubbed BRNN-LSTM. Empirical study shows that BRNN-LSTM achieves a significantly higher prediction accuracy when compared with the statistical approach.
DOI
10.1186/s13635-019-0090-6
Recommended Citation
Fang, Xing; Xu, Maochao; Xu, Shouhuai; and Zhao, Peng, "A Deep Learning Framework for Predicting Cyber Attacks Rates" (2019). Faculty Publications - Information Technology. 2.
https://ir.library.illinoisstate.edu/fpitech/2
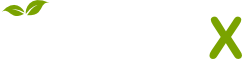
- Citations
- Citation Indexes: 63
- Patent Family Citations: 1
- Usage
- Downloads: 82
- Abstract Views: 4
- Captures
- Readers: 116
Comments
First published in EURASIP Journal on Information Security (2019) 2019:5. https://doi.org/10.1186/s13635-019-0090-6.
This article is distributed under the terms of the Creative Commons Attribution 4.0 International License (http://creativecommons.org/licenses/by/4.0/), which permits unrestricted use, distribution, and reproduction in any medium, provided you give appropriate credit to the original author(s) and the source, provide a link to the Creative Commons license, and indicate if changes were made.
Data used in this work is not suitable for public use. The source code used in the present paper is available at https://github.com/xingfang912/time-series- analysis.